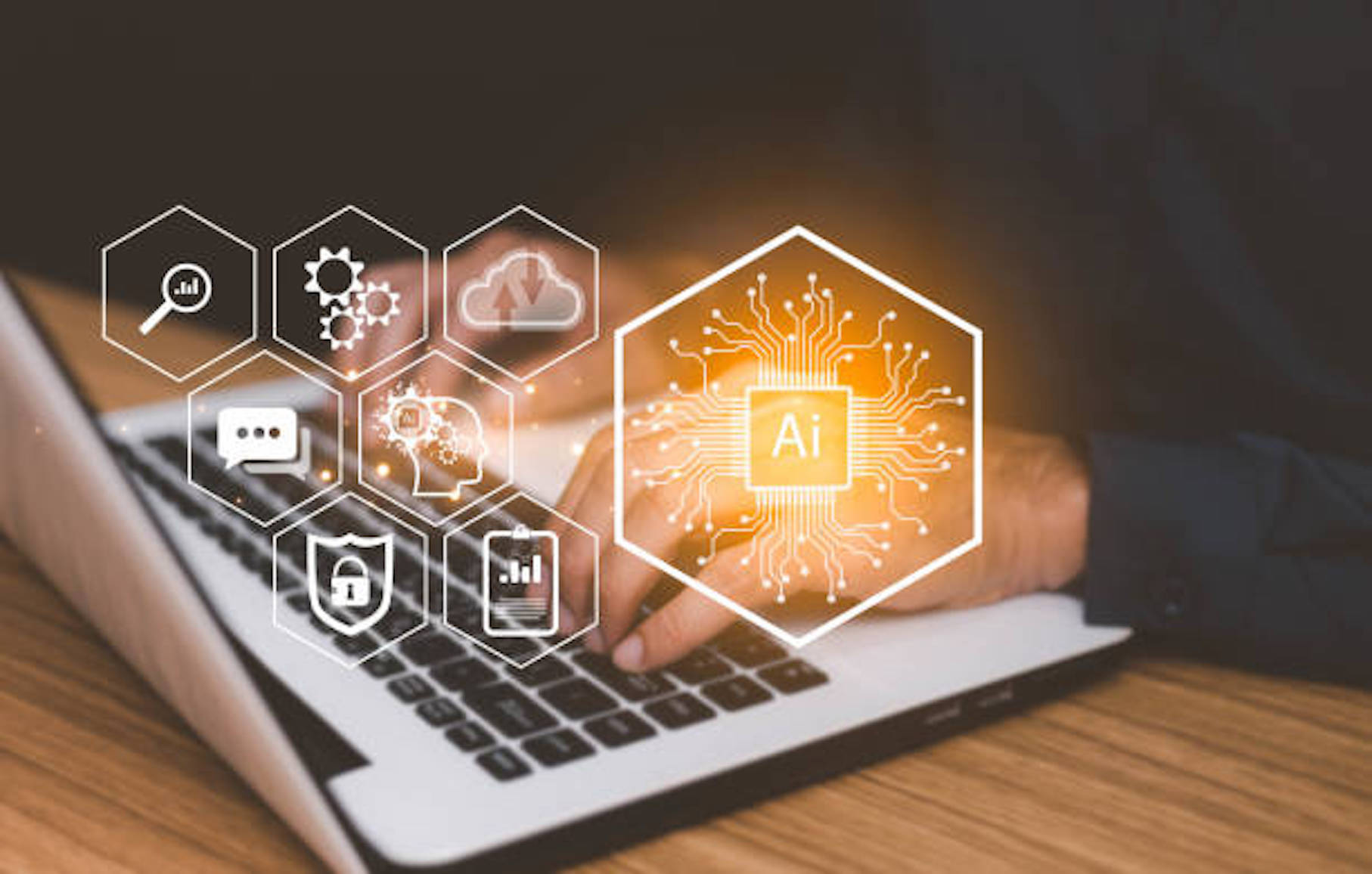
Blogpost \ Technology’s Trojan Horse: The legal issues surrounding algorithmic monitoring of employees at the workplace
Introduction
The monitoring of employees constitutes a phenomenon inherent to humankind, which has accompanied us at least since the industrial revolution. (Aiha Nguyen, 2021)
Monitoring has always been, and always will be, about control: Controlling the work pace, the worker’s dedication, and the worker’s compliance with the work ethic. In that sense, modern monitoring systems come in handy as they are capable of collecting an employee’s data through, for example, fingerprint scanning, video surveillance, fitness apps, body sensors, and many more. (Aiha Nguyen, 2021) However, ever since the introduction of Artificial Intelligence (AI), the monitoring of employees has taken a dark turn. Monitoring has not only become almost entirely automated and perpetuated, but, most importantly, invisible. Especially, the aspect of invisibility represents a significant risk, as employees may unknowingly consent to data collection and privacy violations without being adequately informed about it. Moreover, algorithmic functions often ‘lack the necessary safeguards’ to prevent discrimination, bias, or non-transparency. With other words, employee monitoring has the potential to harm employees in various ways, such as violating their privacy or negatively impacting their mental and physical well-being. (Wilneida Negrón, 2021)
Within the European legal framework, the General Data Protection Regulation (GDPR) forms the fundamental basis for employee protection regarding digital monitoring. Although the GDPR has several shortcomings, the European regulator attempted to fill these existing gaps with the Proposed AI Act, the New Product Liability Directive, and the AI Liability Directive. However, this contribution argues that neither of these new regulations manages to do so. In this contribution, it will not only be pointed out that employee monitoring brings along various risks, but also that the current European legal framework remains to be insufficient to adequately safeguard employees from unethical monitoring.
AI Operation At The Workplace
Using AI at the workplace offers many advantages and has proven itself, in particular, with regard to co-relating, categorising, and controlling of actions or operations. (Antonio Aloisi and Elena Gramano, 2021) The intelligent machines have tremendous processing capabilities, designed to collect or analyse aggregated data by rapidly refining large amounts of information. (Jeffrey Tan, 2017) Thereby, AI operates at lower cost and is less-prone to inefficiency than its human counterparts. AI is better-suited to make objective predictions about the value or success of certain processes. As a result, selection and prioritisation, as well as risk management, identification of possible gaps, and data validation, based on statistics, are handled well by AI (Antonio Nieto-Rodriguez and Ricardo Viana Vargas, 2023) Nonetheless, the question remains, how exactly can companies extract the relevant data for these predictions?
The easiest way to obtain such data is by monitoring the company’s employees. Thereby, workplace monitoring can be conducted in many different ways: This includes monitoring employee productivity, for example via CCTV, location tracking, keystrokes or screen recording software. (Aiha Nguyen, 2021) Indeed, monitoring through algorithmic functions enables employers to guide, evaluate and discipline their employees in ways that cannot be achieved through less-invasive means. (Katherine Kellog, Melissa Valentine and Angéle Christin, 2020)
Other forms of surveillance may include health screening, automated payment systems, or data-centric technology to monitor physical activity and vital signs. (Wilneida Negrón, 2021) (Aiha Nguyen, 2021) This can be done in the most harmless way, such as a company providing its employees with Fitbits to track their steps at work, which, of course, continues to collect data when the employee returns home. As a result, such an examination often blurs the line between the clear separation of work and personal life.
Monitoring can also be done through location tracking or behavioural analysis. For example, Uber tracks its employees using GPS, while other employers use AI for automated hiring, in which an algorithm analyses a candidate’s facial expressions, vocabulary, or voice to create a ranking system. (Jeremias Adams-Prassl, 2022)Employee monitoring can also be carried out with the help of third parties, for example in the food industry.(Karen Levy, 2018) Customers create and submit reviews that serve as a reference for an employee’s working hours. These are then evaluated and interpreted by an algorithm, which, in the worst case, can even lead to the termination of the employee’s employment. (Carolien O’Donovan, 2018)
Associated Risks
At the core of employee monitoring lies the issue that employers are often voraciously intent on increasingprofits, decrease expenses, safeguard assets, and maintain oversight over work processes and productivitylevels. Subsequently, employers ignore workers’ rights and needs, which eventually leads to harming them.
Being aware of monitoring mechanisms can greatly affect an employee’s sense of job quality, psychological well-being, or trust in the employer. It can also limit the concept of work-autonomy, resulting in lower work efficiency. (Sara Riso, 2020) Nonetheless, employee monitoring through algorithmic functions brings along even greater risks, such as exploitation, a lack of transparency due to its non-deterministic nature, bias and discrimination, or data protection violations.
Exploitation
Utilising AI systems to monitor employees brings about a fundamental shift in power dynamics. (Sara Riso, 2020) For example, the introduction of opaque pay models, known as ‘black-box’ systems, facilitates practices such as wage-fixing or no-poaching, leading to significant obstacles for employees in finding better job opportunities in the long run. (Wilneida Negrón, 2021) Initially, this can result in economic exploitation of employees, encompassing wage theft, miscalculation, or suppression.
Moreover, this phenomenon is closely linked to the exploitation of workers in low-wage industries, as those heavily reliant on their income are more likely to endure unfair working conditions, including algorithmic monitoring, out of fear of losing their jobs. Consequently, working conditions that involve constant monitoring not only foster heightened competition among employees, but also create an atmosphere resembling a game, which prevents employees from forming alliances against their employers. (Sara Riso, 2020) As a result, employees experience dissatisfaction, increased work intensity, anxiety, and overall diminished well-being.
Non-determinism
The implementation of employee monitoring and tracking, including the use of performance-based scoring systems, can result in excessive disciplinary measures. (Sara Riso, 2020) These scoring systems are susceptible to algorithmic manipulation, where the AI can alter its response mechanisms based on the specific context it operates in, making it challenging to control. With other words, the AI’s behaviour becomes non-deterministic, generating different responses for each instance of execution without providing clear insight into the factors causing these variations. Consequently, it seems unclear whether the algorithmic system may misinterpret an employee’s performance. Thus, if employers heavily rely on the AI’s findings, thiscan lead to negative consequences which may significantly impact the employee. (Antonio Aloisi and Elena Gramano, 2021)
Bias and discrimination
Algorithmic systems, being shaped by the ethics of their creators, develop in a non-linear fashion whilst lacking awareness of causality. This means, that these algorithms are prone to generating biased or discriminatory outcomes, particularly affecting vulnerable individuals such as immigrants, women, the elderly, or those with physical disabilities in the workplace. Due to physical limitations or language barriers, their performance may be unfairly deemed lower compared to other workers, even though the AI fails to establish a causal connection between these factors. (Antonio Aloisi and Elena Gramano, 2021)
This form of discrimination is often categorised as ‘indirect discrimination’, allowing employers to invoke justifications based on proportionate reasoning or legitimate aims. (Jeremias Adams-Prassl, 2022) In particular, victims of proxy discrimination often face significant challenges in the legal realm, as the law seeks to prohibit discrimination based on traits that ‘contain predictive information not directly captured by non-suspect data’. (Philipp Hacker, 2018) Thereby, proxy discrimination refers to situations where the model cannot directly capture certain traits and relies on proxies instead. (Aude Cefaliello and Miriam Kullmann)
The stance of the European Court of Justice on whether biased algorithmic decision-making can be considered ‘direct discrimination’, remains unclear. However, it is likely that if the affected individual belongs to a protected group with ‘absolute and unconditional priority’, the answer, establishing discrimination, would lean towards affirmative.
Data Protection
AI systems introduce multiple information asymmetries, resulting in decision-making that is both incomprehensible and unpredictable. This lack of transparency poses challenges in protecting the data of employees. In reference to Article 7 of the GDPR, which emphasises the importance of ‘informed consent’, it becomes difficult to fully assess risks when the opacity of data processing hinders comprehension. Monitoring takes place in an automated and continuous manner, often without the employee’s awareness, making it challenging to meet the threshold of informed consent. (Kirstie Ball, 2010) Especially, if an employee signs a consent form that grants the employer access to their data, they effectively relinquish control over how that data is collected and utilised. (Aiha Nguyen, 2021) Furthermore, negotiations regarding the scope of consent are frequently denied, raising concerns about the fairness of such consent forms, since employees are unlikely to refuse data collection if the alternative is unemployment.
Additionally, collected data is not limited to a single purpose but can be repurposed, used for AI training, and likely retained within the company’s database even beyond the employee’s tenure. (Jeremias Adams-Prassl, 2022) Storing vast amounts of sensitive data within a company’s database also increases the risk of data abuse. Unfortunately, employees often remain unaware of potential infringements on their rights, further exacerbated by the absence of adequate safeguards. (Aiha Nguyen, 2021)
The Current Legal Framework And Its Shortcomings
The GDPR
The GDPR functions as a general instrument for basic data protection rights, which also includes the rights of employees. However, the GDPR seems to adopt an outdated perspective towards emerging technologies, as it fails to address the various ways in which data can be utilised or misused in the context of novel algorithmic functions. (Antonio Aloisi and Elena Gramano, 2021) The Regulation takes a narrow approach, primarily focusing on data collection while neglecting the risks associated with data analysis. This includes inferential analytics, which involves drawing conclusions based on random data through ‘correlative patterns’ or logical deductions. (Sandra Wachter, 2018)
Additionally, the GDPR’s conceptualisation of data is limited, as it fails to account for new market strategies and solely concentrates on profiling. (Antonio Aloisi and Elena Gramano, 2021) It is important to note,that the technology industry is fundamentally reshaping the public services market by utilising algorithmic monitoring as a form of market power, which this regulation fails to address
The Proposed AI Act
The Proposed AI Act introduces a distinct approach to regulating AI, specifically by prohibiting practices like ‘social scoring’ and ‘biometric identifications’. However, the criteria outlined in the Act do not appear to be stringent enough. For instance, the responsibility for assessing the conformity of ‘high risk’ AI systems to guarantee ‘safe usage’ is left to employers rather than relevant authorities. This raises concerns about the efficacy of oversight and accountability.
Furthermore, it almost seems as if AI is perceived as a regular machine, rather than a complex technology. This classification fails to address or adequately apply to the multitude of risks associated with AI systems in the workplace. (Aude Cefaliello and Miriam Kullmann) Regrettably, the AI Act does not establish sufficient connections with the existing legal instruments that are essential for protecting employees effectively.
The New Product Liability Directive And The AI Liability Directive
The New Product Liability Directive, on one hand, aims to expand the scope of ‘products’ to encompass AI systems. Consequently, compensation for damages caused by AI systems would be treated on par with damages caused by any other product involving human action. Hence, this Directive represents a groundbreaking step in attributing fault-based liability and imposing obligations for reparations in relation to actions carried out by AI systems.
The AI Liability Directive, on the other hand, establishes a framework for addressing damages caused by high-risk AI systems through non-contractual civil liability and the submission of supporting evidence. It is encouraging to observe that the regulator acknowledges and seeks to mitigate the black-box effect associated with AI systems through this Directive. (Ioana Bratu, 2023) Particularly, the Directive highlights the lack of transparency inherent in AI systems. However, it falls short in specifying appropriate alternatives for submitting causal evidence in case of such transparency issues.
Concluding Remarks
The significance of AI in the workplace is on the rise, yet the associated challenges remain inadequately addressed. These challenges encompass privacy violations, potential harm to physical and mental health, and more. A critical issue concerning employee monitoring is the lack of awareness among workers regarding their rights and the infringements that may occur. To this end, practicing lawyers should not rely on the good intentions of employers, but rather focus on the many risks that can arise from the implementation of employee monitoring. (Sara Riso, 2020)
While the existing legal framework, including the GDPR, offers some level of employee protection, significant gaps persist. For instance, the issue of consent-based data processing remains problematic in employer-employee relationships, as genuine consent is rarely achievable. Employees often remain unaware of the extent of data processing, their contribution to data, and the factors influencing decisions, making the notion of ‘given-consent’ nearly impossible. (Sara Riso, 2020) Also the proposed AI Act, AI Liability Directive, and New Product Liability Directive still fall short in certain areas, although they represent steps into the right direction.
It is crucial to establish proactive regulations that effectively address the multitude of issues arising from employee monitoring, whilst also relieving workers from the burden of taking individual action. Holding employers accountable and enabling employees to seek damages for serious infringements of their rights caused by AI monitoring should be easily accessible and enforceable. In conclusion, achieving effective regulation of AI in the workplace requires striking a balance between the advantages of technology and the imperative to respect workers’ rights, ensuring ethical and transparent use of AI.